Seeing Past the AI Hype
Artificial intelligence won’t take your job—but what are the real concerns around healthcare AI and its use?
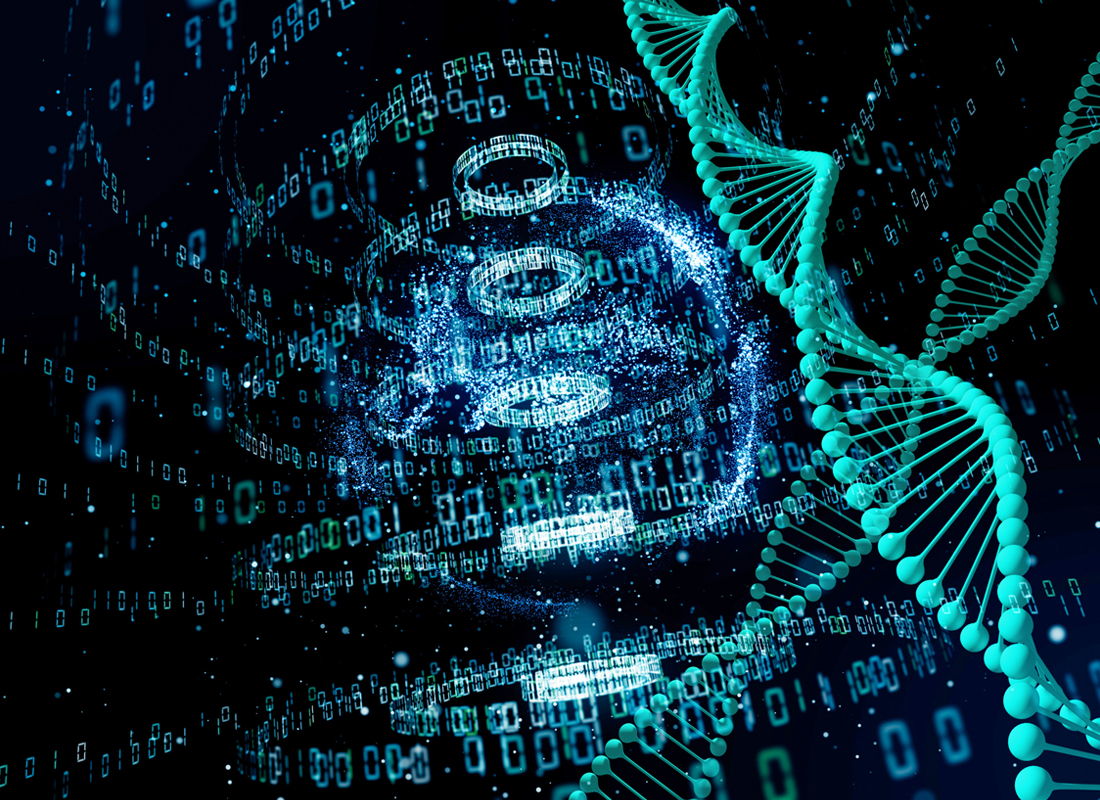
As artificial intelligence (AI) advances in healthcare, so does its hype. “Learn AI now or risk losing your job!” headlines declare as news media highlight algorithms’ ability to increase diagnostic accuracy, discover new biomarkers, and even generate clearer reports. It’s no wonder that 44 percent of healthcare professionals fear AI may pose a threat to their jobs1—but is this justified?
“At least in my lifetime, that won’t be possible,” says digital and computational pathology expert Rajendra Singh, MD, co-founder of PathPresenter and director of dermatopathology at Northwell Health. “It might happen in the next few hundred years, but it’s still a long way off.” Instead of fearing job losses, Singh urges laboratorians and administrators to learn more about AI, how it can enhance their work, and the real concerns that must be addressed before it can enter routine clinical use.
Validation is vital
“Whenever a new AI model is produced, each institution needs to validate it with their own data,” Singh says. “You cannot rely on information gathered by a different laboratory under different conditions.” This means that labs who want to take full advantage of emerging AI technologies should start thinking now about how to validate the models they use. What processes will be involved? What data will be required? What protocols will need to be implemented?
Singh also highlights the challenge regulatory approval poses in a rapidly changing AI environment. “How can we expect regulatory organizations to validate every AI model and every update?” New algorithms arise almost daily, and existing ones are regularly updated, making it impossible for any regulatory body to keep pace—so approvals would essentially have to “lock” algorithms, preventing further development or improvement. But Singh has an alternative suggestion. “Developing and approving rigorous validation protocols might be much more practical than trying to validate each algorithm individually,” he says.
Finding the funding
For administrators, a key concern around AI is who pays for the technology. Singh says, “I think that has been a major stumbling block because there are very few Current Procedural Terminology (CPT) codes for AI-based diagnostic support—but I think that will change in the next few years as insurance companies begin seeing its value in the diagnostic process.”
In fact, CPT codes have already begun changing in response to the evolution of healthcare AI. Since January 2022, CPT Appendix S: Artificial Intelligence Taxonomy for Medical Services and Procedures2 has been in effect. The taxonomy recognizes three categories of AI: assistive (detecting clinically meaningful data), augmentative (analyzing or quantifying data in clinically meaningful ways), and autonomous (interpreting data and drawing clinically meaningful conclusions).3
But even AI that performs autonomous tasks is not truly independent. Some “autonomous” algorithms require a human laboratorian to select from a range of treatment options, approve recommended treatment options, or override incorrect or uncertain conclusions. Algorithms that can complete all of these steps without human intervention still require oversight. “The most important concern is: who is responsible for the AI’s result?” says Singh. “I believe it will always be the clinician. Nobody else can sign off that the result is accurate—or at least as likely to be accurate as a human-produced diagnosis.”
Protecting patient privacy
Patient privacy is a vital consideration in any medical setting, but building healthcare AI models requires patient data. So how do you ensure adherence to ethical principles4 and privacy standards? “Right now, data are often sent to an external server, because AI providers don’t put their models on local institutional servers,” Singh cautions. “So, when we share data to build or validate AI models, we have to be very careful—first, that we are providing only data we have the patient’s permission to share, and second, that we provide it as securely as possible.”
Singh highlights the rise in generative AI-related privacy concerns. “It’s only in the last year or so that people have started uploading healthcare data to test these models—and they often forget that any data put into the model becomes part of the data used to train it. It’s very important to make everybody aware that any data fed into a generative AI model may become visible to others. No data leaving the institution should include protected health information that could link back to a patient.”
Hallucinations and déjà vu
Generative AI models may invent “hallucinations”—results or references that don’t exist in the real world.5 They may also be subject to the “déjà vu” phenomenon, which diagnosticians may recognize; one example is when diagnostic AI generates results based not on the pathological entity on a slide, but on its background.
“Right now, if you test some of the latest generative AI models with images or information, they will always give you an answer,” says Singh. “The fact that they never say that they don’t know or recommend consulting an expert is very scary.” He believes that companies building such models need to set limitations on them so they cannot generate inaccurate or unverifiable results. One way to achieve this might be to implement a threshold value for response accuracy or similarity; if no results exceed that threshold, the AI model would simply report that it has no response.
“Precautions like these will be critical to the future of AI, especially in highly sensitive applications such as healthcare,” says Singh, who has encountered both hallucinations and déjà vu in his own practice. “We’ve seen that, if you provide a large language model with images and then ask 10 diagnostic questions, five or six of the answers will be highly accurate. The remainder might be low-quality or entirely inaccurate. That worries me because experienced laboratory medicine professionals may recognize incorrect responses, but medical students and residents may not. And if that inaccurate information is used for patient diagnosis or treatment, it could cause serious problems.”
An eye to the future
Singh thinks it’s vital for everyone involved in pathology and laboratory medicine to understand exactly what AI can do—and what it can’t. “I want people to understand that these models are still very narrow and have significant limitations. Physicians base their conclusions on the full context of the patient’s history and clinical picture—not just a single histology slide or radiology image. AI models have intelligence, but lack common sense and ‘gut feelings.’ Although they can effectively identify specific characteristics or predict specific outcomes, an AI model that can handle a task as broad as ‘help me make a diagnosis’ is very far away at the moment.”
References:
- Commins J. AI feared as job snatcher by nearly half of healthcare workers. HealthLeaders. March 10, 2023. https://www.healthleadersmedia.com/technology/ai-feared-job-snatcher-nearly-half-healthcare-workers.
- CPT Appendix S: Artificial Intelligence Taxonomy for Medical Services and Procedures. American Medical Association. August 12, 2022. https://www.ama-assn.org/system/files/cpt-appendix-s.pdf.
- Frank RA et al. Developing current procedural terminology codes that describe the work performed by machines. NPJ Digit Med. 2022;5(1):177. doi:10.1038/s41746-022-00723-5.
- Ethics and Governance of Artificial Intelligence for Health. World Health Organization. June 28, 2021. https://iris.who.int/bitstream/handle/10665/341996/9789240029200-eng.pdf.
- Hatem R et al. A call to address AI “hallucinations” and how healthcare professionals can mitigate their risks. Cureus. 2023;15(9):e44720. doi:10.7759/cureus.44720.
Subscribe to Clinical Diagnostics Insider to view
Start a Free Trial for immediate access to this article