Removing Racial Inequalities in Lab Referrals
Clinical laboratory professionals must consider how race affects test referrals and what they can do to combat existing inequities
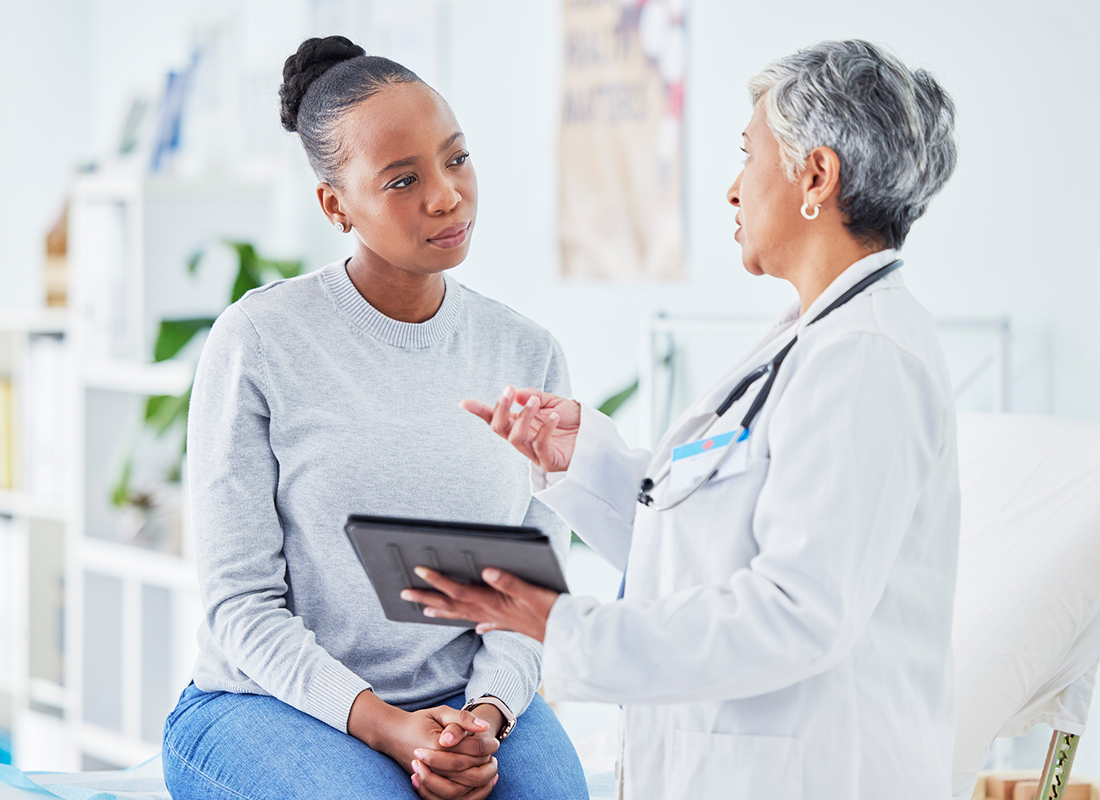
Successfully navigating the intersection of race and medicine is a nuanced task made even more complex by a lack of consensus regarding the clinical relevance of race.1 Arguments in favor of race-based medicine claim that the clinical value of racial and ethnic categories can be found in their ability to reflect underlying population genetics; those against view race as a sociopolitical construct founded on transient factors and therefore lacking in clinical accuracy and utility. In such a polarizing landscape, overshadowed by a well-documented history of racism,2 clinical laboratory professionals must consider how race affects test referrals and what they can do to combat existing inequities.
Decisions of many factors
Do primary care physicians’ (PCPs) specialist referral patterns differ based on a patient’s race? Although there has been little research directly dissecting the effect of race on test orders, a 2021 study on PCP referrals of Black and White Medicare beneficiaries in 12 areas across the US suggests concerning differences.3 Looking at referrals to a range of specialties, the researchers found that the number of specialists seen by a patient within an episode of care was lower for Black patients than for White patients. The gap narrowed, but still persisted, when the White patient population was sampled to equalize the number of Black and White patients seen.
Differing referral rates between racial groups, whether to the clinical laboratory or elsewhere, isn’t necessarily the result of explicit bias. Instead, they may arise from the inclusion of race within contemporary treatment algorithms, laboratory equations, diagnostic criteria, screening recommendations, and other clinical decision-making processes.4 Such race-based considerations are used across the scope of medical specialties, as illustrated by a partial list compiled by researchers in 2020.5 They highlighted, for instance, that the urinary tract infection (UTI) calculator, which estimates UTI risk in children under two years, reports an approximately 2.5-fold higher likelihood of UTI in non-Black patients. The systematic lower reported risk of UTI in Black children may dissuade clinicians from pursuing definitive diagnostic urine testing for this population, even when patients present with symptoms of UTI. A 2022 study demonstrated the potential negative effects of such algorithms by revealing significant differences in screening and referral practices for nonalcoholic fatty liver disease (NAFLD) by race/ethnicity; only 17 percent of African American pediatric patients with abnormal liver enzymes were referred for screening labs, compared with 87 percent of non-Hispanic White and 92 percent of Hispanic White patients.6
AI: benefit or bias?
Artificial intelligence (AI) tools are increasingly being implemented as part of the diagnostic process—and are helping healthcare professionals identify unencoded or rare diseases in undiagnosed and under-diagnosed patients.7 However, despite their potential, AI systems have also been shown to exhibit racial bias, which can perpetuate or even amplify pre-existing biases in medicine.8 This was demonstrated in a 2019 study that evaluated the racial bias of an algorithm used to predict the healthcare needs of approximately 200 million Americans.9 The researchers found that Black individuals were assigned lower risk scores than their White counterparts and, consequently, had to be much sicker before receiving referrals for extra care. Only 17.7 percent of those referred for additional care were Black, far lower than the 46.5 percent that the researchers calculated would have been referred using an unbiased algorithm.
Responding to growing concern, the Agency for Healthcare Research and Quality (AHRQ) and the National Institute on Minority Health and Health Disparities (NIMHD) has assembled a diverse expert panel to identify steps for eliminating racial algorithmic bias in healthcare.10 “As the use of new AI techniques grows and grows, it will be important to watch out for these biases to make sure we do no harm to specific groups while advancing health for others. We need to develop strategies for AI to advance health for all,” explained panel co-chair Lucila Ohno-Machado, MD, PhD, MBA.11
A role in referrals
What can laboratorians and administrators do to combat racial inequities in the referrals they receive? The clinical lab’s role in educating PCPs cannot be understated. Educational strategies and interventions such as lectures, guidelines, and clinical decision support system updates have been effective in consistently driving change—for instance, by helping physicians optimize laboratory test utilization.12 Alongside this, laboratory professionals can collaborate with PCPs to address racial inequalities from patient care before, during, and after the lab’s involvement. An example is the elimination of race as a proxy in kidney function calculations in the NYC Health + public health system, the result of a partnership between NYC Health + Hospitals Nephrology Workgroup and Laboratory Council as part of their “Medical Eracism” initiative.13
Such collaboration requires deep analysis of contemporary practices to identify the racialized biases that are ingrained in the status quo and remove or correct those that are medically and statistically significant.14 To fully engage with this analysis, laboratorians should raise questions about racial bias where necessary, assess the potential biases of technologies they use or plan to adopt (and how those biases can be avoided or corrected), explicitly eliminate bias in the design of laboratory-developed tests, and develop policies to nullify the impact of bias.
A recent review shows that there’s no one-size-fits-all way to re-evaluate algorithms.15 Even those that don’t explicitly use race can be implemented in ways that affect racial disparities—whether positively or negatively—potentially leading to unintended consequences. For example, removing race in calculations of estimated glomerular filtration rate improved the accuracy of kidney disease diagnosis and classification in Black patients, potentially leading to broader and earlier eligibility for kidney transplants—but the change also risked reducing those patients’ access to chemotherapy, enrollment in clinical trials, and dosing of antibiotics, highlighting the need for reforms to be more than skin deep.
Counteracting bias in medicine, particularly when it prevents patients from receiving the tests, diagnoses, and care they need, is not an easy task. But, as NYC Health + Hospitals senior vice president and chief quality officer Eric Wei said in a press release, it is vital to achieving equitable care for all patients.13 “Calculating medical conditions can be a matter of life or death in most cases. By eliminating some simple biological tests, we are helping to eliminate an element of systemic racism in medicine and sending our communities a message of how seriously we take the health and wellbeing of all of our patients.”
References:
- Manski CF et al. Using measures of race to make clinical predictions: decision making, patient health, and fairness. Proc Natl Acad Sci USA. 2023;20(35):e2303370120. doi:10.1073/pnas.2303370120.
- Duello TM et al. Race and genetics versus ‘race’ in genetics. Evol Med Public Health. 2021;9(1):232–245. doi:10.1093/emph/eoab018.
- Landon BE et al. Assessment of racial disparities in primary care physician specialty referrals. JAMA Netw Open. 2021;4(1):e2029238. doi:10.1001/jamanetworkopen.2020.29238.
- Kaufman JS et al. Use of racial and ethnic categories in medical testing and diagnosis: primum non nocere. Clin Chem. 2021;67(11):1456–1465. doi:10.1093/clinchem/hvab164.
- Vyas DA et al. Hidden in plain sight – reconsidering the use of race correction in clinical algorithms. N Engl J Med. 2020;383(9):874–882. doi:10.1056/NEJMms2004740.
- Patil R et al. Screening and referral practices for nonalcoholic fatty liver disease by race and ethnicity in a primary care clinic. J Racial Ethn Health Disparities. 2023;10(3):1456–1465. doi:10.1007/s40615-022-01324-4.
- Nia NG et al. Evaluation of artificial intelligence techniques in disease diagnosis and prediction. Discov Artif Intell. 2023;3(1):5. doi:10.1007/s44163-023-00049-5.
- Mittermaier M et al. Bias in AI-based models for medical applications: challenges and mitigation strategies. NPJ Digit Med. 2023;6(1):113. doi:10.1038/s41746-023-00858-z.
- Obermeyer Z et al. Dissecting racial bias in an algorithm used to manage the health of populations. Science. 2019;366(6464):447–453. doi:10.1126/science.aax2342.
- Chin MH et al. Guiding principles to address the impact of algorithm bias on racial and ethnic disparities in health and healthcare. JAMA Netw Open. 2023;6(12):e2345050. doi:10.1001/jamanetworkopen.2023.45050.
- Forman R. Eliminating Racial Bias in Health Care AI: Expert Panel Offers Guidelines. December 21, 2023. https://medicine.yale.edu/news-article/eliminating-racial-bias-in-health-care-ai-expert-panel-offers-guidelines/
- Lillo S et al. The impact of interventions applied in primary care to optimize the use of laboratory tests: a systematic review. Clin Chem Lab Med. 2021;59(8):1336–1352. doi:10.1515/cclm-2020-1734.
- NYC Health + Hospitals. “Medical Eracism” Initiative Aims to Abolish Race-Based Assessments Used for Medical Decisions. May 17, 2021. https://www.nychealthandhospitals.org/pressrelease/medical-eracism-initiative-aims-to-abolish-race-based-assessments-used-for-medical-decisions/
- Coskun A. Bias in laboratory medicine: the dark side of the moon. Ann Lab Med. 2024;44(1):6–20. doi:10.3343/alm.2024.44.1.6.
- Siddique SM et al. The impact of health care algorithms on racial and ethnic disparities: a systematic review. Ann Intern Med. 2024; online ahead of print. doi:10.7326/M23-2960.
Subscribe to Clinical Diagnostics Insider to view
Start a Free Trial for immediate access to this article