Using AI to Improve Reimbursement and Decrease Claim Denials
Though payers’ adoption of AI has made reimbursement more challenging for labs, providers can also use AI to address these issues
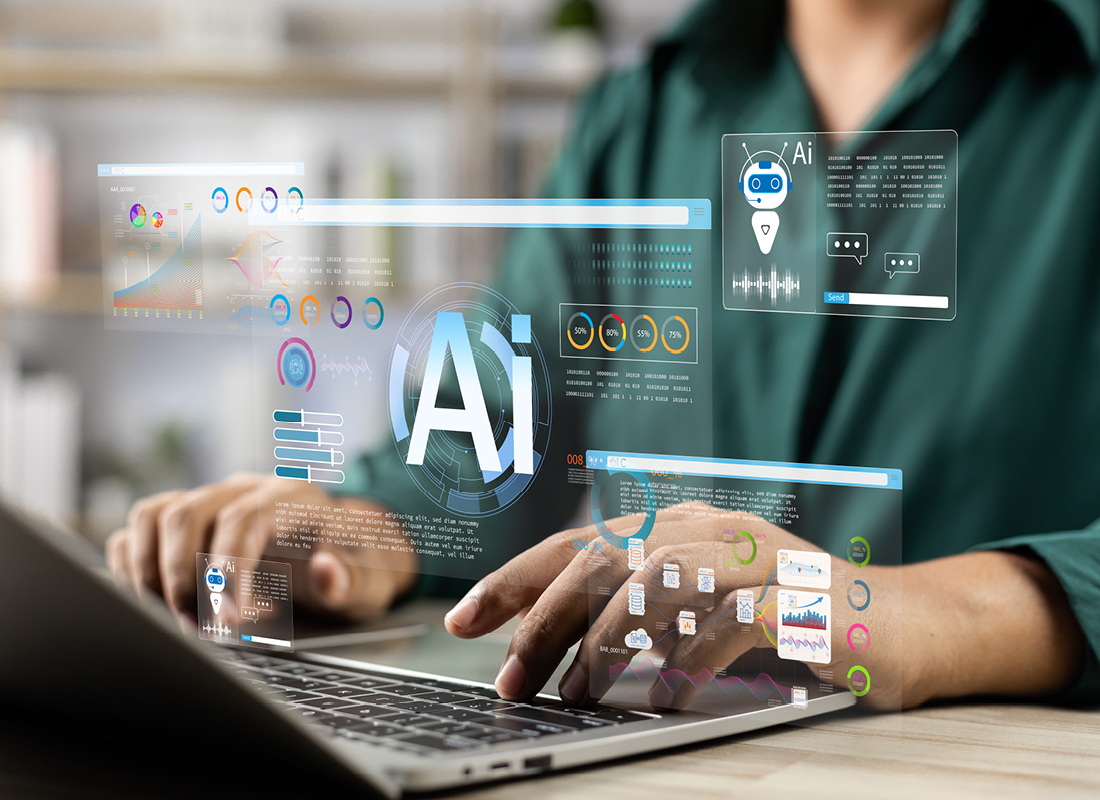
Artificial Intelligence (AI) is everywhere. Entities that can harness AI capabilities to boost performance have welcomed this innovation, including payers. Laboratories can also benefit from AI, improving reimbursement and decreasing claim denials by adopting this technology in the right fashion.
As one example, laboratory billing practices can be optimized by using AI. This is especially important, as payers are adopting AI to more efficiently process claims. Learning how to properly employ revenue cycle management (RCM)-specific AI in your lab can help improve the stability of your lab’s economic standing through enhanced reimbursement, fewer claim denials, and reduced labor costs.
Understanding payer AI use
The volume of claims that payers review is extensive. To maintain accuracy and efficiency in their operations, which have a direct impact on the patients they insure, payers have begun employing AI to more quickly process claims.
Most private payers have specific policies determining what services can be reimbursed, and have now adopted AI to automate claim reviews. AI is trained on the rules for reimbursement approval and denial. The technology is then able to review claims to ensure all necessary information is provided and the guidelines for reimbursement are met. If information is missing or does not meet reimbursement standards for any reason, AI will then deny that particular claim. The denial can be appealed, of course, but not without additional time and attention from a lab’s billing staff, which negatively impacts efficiency and potentially delays patient care. In our September 2024 Lab Industry Advisor issue, we discussed how understanding and adhering to each payer’s policies regarding the process for reimbursement is necessary in order for labs to optimize reimbursement outcomes and avoid denials.1
In addition to establishing that claims meet all requirements ahead of issuing reimbursement, payers are also considering using AI to deny claims that are suspicious in hopes of detecting and decreasing Medicare fraud.2 The U.S. Department of Health and Human Services (HHS) estimated that over $100 billion in improper payments were issued by federal programs during fiscal year 2023.3 Use of AI could be a big help in addressing such payments. However, it also means that, should your lab not follow the payer’s specific policies, the claim, even if legitimate, may be flagged by AI and denied for reimbursement, something providers are increasingly taking issue with.
For example, Humana, Cigna, and UnitedHealthcare are facing class action lawsuits for allegedly using AI to deny claims. The lawyers overseeing the suit have interviewed dozens of Medicare policyholders and physicians who are frustrated with the technology denying claims for services. Providers and patients feel as though payers are implementing AI under the guise of faster claim processing while enjoying the increase to their bottom line resulting from these increased claim denials.4
Payers have been taking advantage of AI to help streamline their processes and speed decision-making, but in many cases, they are effectively using AI to deny claims in several ways, as seen in the jump in denial rates from 10 percent in 2020 to nearly 12 percent at the end of 2023.4 The increase in claim denials is due to many factors, including:
-
- automation of the claim review process,
-
- denial of claims that do not align with preset medical necessity criteria,
-
- higher prior authorization denials due to a less rigorous AI review process as compared to human review,
-
- the utilization of AI specifically to decrease payer costs,
-
- the use of AI review to bypass the physician’s role in the claims review process (which also decreases payer costs), and
- the rigidity of AI-driven decisions.
Insurers, however, state they don’t use AI to make coverage decisions.4 According to Jeff Carmichael, senior vice president of engineering and AI at XiFin, payer responses are often complicated to address, even when they don’t use AI. This complexity has made providers’ use of the technology essential.
“It is now critical that AI is used in RCM processes to ensure that patients are not held responsible for the portion of their healthcare that another party should pay and that labs can be paid for the vital services they have already provided,” Carmichael explains.
Providers use of AI to understand claim denials
To combat payers’ use of AI and increased claim denials, providers have begun to adopt the technology in order to understand denials and to better shape their billing processes to decrease such denials.5
Luminis Health has launched automation and machine learning to ensure their claims are accurate ahead of submission to payers. Mayo Clinic has saved $700,000 in vendor costs and eliminated 30 full-time employee positions by employing AI bots in their RCM department. The medical center has increased efficiency and productivity by building bots that can handle tasks such as checking the status of claims, closing duplicate denials, redacting documents, and monitoring prior authorization status. In another example, Care New England has seen a 55 percent reduction in claim denials related to authorization by using bots to help handle payer notification when patients are admitted to the hospital, a process that can be administratively tedious due to the varying requirements between payers.5
Vendors in the healthcare RCM space are helping, too. In response to the challenge posed by tens of thousands of unstructured responses from payers on claims paperwork, for example, XiFin has tested a new payer response translation AI. This tool consumes unactionable payer messages, translates, and maps them to an actionable code. “The results of the pilot are exceedingly positive,” Carmichael says.
How your lab can effectively employ AI
There are several key challenges faced by labs due to payer integration of AI. Some of these include delayed reimbursement payments, higher administrative burden, barriers designed to make reimbursement more difficult to obtain, inadequate physician review, and strained relationships between providers and payers, as well as the increase in denials.
However, AI can also help labs, particularly when it comes to denials related to medical necessity.
“We are beginning to see an emergence of AI tools that can data mine the patient’s medical records to create [a] highly specific statement of medical necessity within a patient’s chart,” explains Dyana Williams, director of RCM consulting at Lighthouse Lab Services. With reimbursement hinging on the clinical necessity of the lab test being ordered, harnessing AI to ensure patient information is accurate and medical necessity is clearly demonstrated should help decrease denials.
Carmichael further emphasizes that AI can be introduced in several ways to help reduce administrative burden while improving reimbursement outcomes by using it to:
-
- Uncover additional payer plan details up front, thereby reducing the need for calls with the patient and reducing impact on call centers.
-
- Evaluate recent remittance advice to more accurately determine expected out-of-pocket expenses and then communicate that estimate to the patient.
-
- Derive accurate predictions, such as expected allowable reimbursement amount, estimated copay, estimated coinsurance, risk of coverage limitations, etc.
- Enable RCM workflows that prioritize and assign exceptions to billing team members. Exceptions can be prioritized based on likelihood of payment and can be rerouted to the best available team member or group.
AI can help improve your lab’s likelihood of reimbursement
Adopting AI in the right way can assist your billing team by potentially decreasing your lab’s claim denials. But one thing many experts point to is that AI is only as smart as the data on which it is trained. A big piece of advice to follow ahead of implementing AI in your lab: proactively clean up your data. “Dirty data leads to unintelligent AI,” Carmichael says. “It’s important to have RCM data expertise in your organization or to work with an RCM partner that has a proven track record in RCM data readiness for optimal AI.”
Finally, keep in mind that AI use will not solve all of your reimbursement issues, and it cannot currently replace your entire billing team. Resources will also need to continue to be devoted to AI development and maintenance, experts say. Though AI cannot solve all billing and reimbursement issues alone, it can presently be used as a tool to increase reimbursement success and decrease claim denials.
References:
Subscribe to view Essential
Start a Free Trial for immediate access to this article