Dissecting the Data Behind Healthcare AI
How do the underlying datasets affect artificial intelligence tools’ performance in the lab—and beyond?
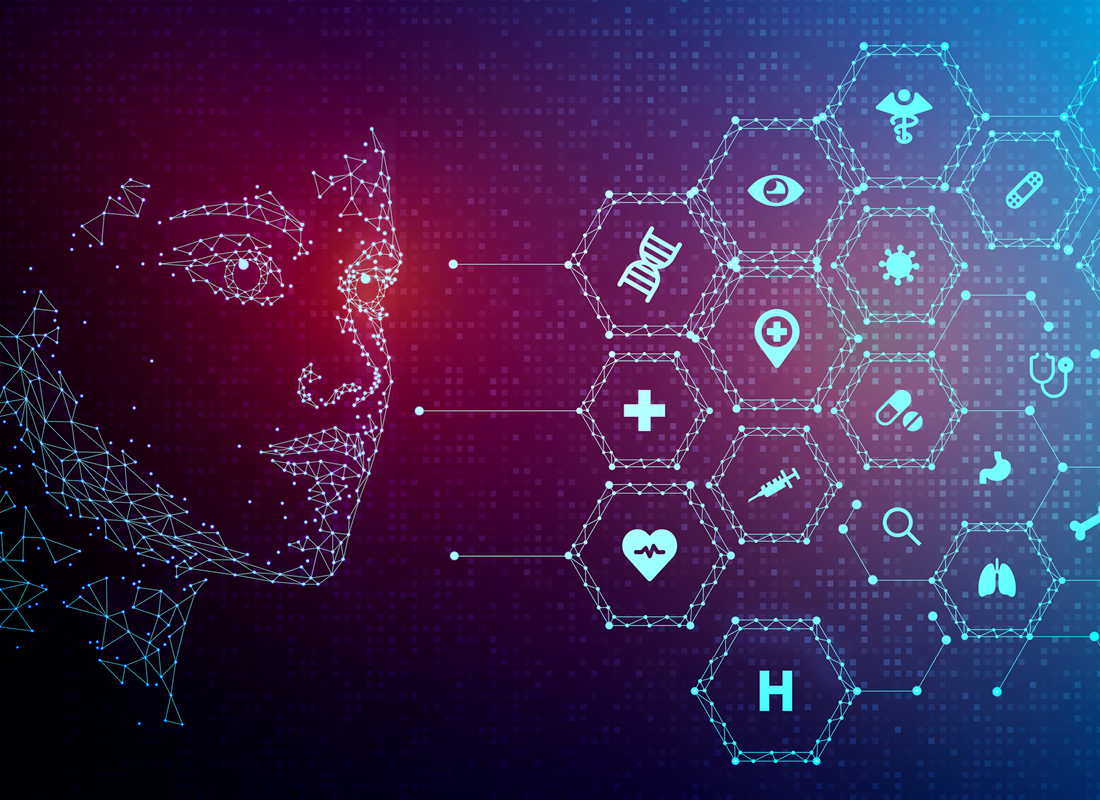
As we enter further into the age of artificial intelligence (AI), this novel technology brings with it new potential across the scope of medicine.1 However, with this potential comes the concern that algorithmic performance may differ between subgroups for predictive tasks—potentially displaying, perpetuating, or even compounding pre-existing health inequalities that arise from socioeconomic status, race, ethnicity, religion, gender, disability, or sexual orientation.2 For example, earlier this year, a Massachusetts Institute of Technology (MIT) research team identified that the AI models most capable of discerning a patient’s race via medical imaging also displayed the biggest fairness gaps, leading to incorrect diagnostic evaluations for women, Black people, and other subpopulations.3 But what exactly causes this bias? How is the performance of AI tools in the clinical lab affected by the data on which they are trained? And what can laboratorians do to mitigate biases and ensure efficacy of their AI training datasets?
Bias in, bias out
“Healthcare data is complex,” explains Leo Celi, clinical research director and principal research scientist at the MIT’s Laboratory for Computational Physiology. Because the datasets used to train, test, and validate AI algorithms are the backbone of the tools’ performance, developers might first look there for answers—but, in doing so, potentially miss bias in the upstream stages of AI development: research problem formulation, data collection, and data preprocessing.4
“When it comes to the data used to develop prediction algorithms, there is what we call the social patterning of the data collection process,” Celi explains. “An example of relevant social patterning might be the people who have access to health care, which in turn drives who gets into the database. We also collect data at different ‘intensities’ across patients in a manner influenced by a host of factors that are not fully understood. Data that developers understand is the building block of AI, but most AI developers are oblivious to the social patterning of the data generation process in health care.” These upstream sources of complexities can reduce developers’ understanding of exactly what informs their algorithms.
Embracing high-quality ‘dirt’
“Data is the backbone of any AI model, so it’s crucial for affecting AI algorithms’ performance,” agrees Michael Moor, assistant professor for medical AI at the Swiss Federal Institute of Technology Zurich. Knowing this, medical AI developers and users alike may want their models trained on as much data as possible; however, Moor explains that simply providing more data isn’t necessarily a recipe for success. “In the healthcare setting, dataset quality is almost more important than quantity—a lot can already be achieved with mid-sized, high-quality datasets.”
What is a high-quality dataset? Although the data needed to train different models to perform different tasks will vary in content, some features should be consistent between datasets. First, like in the upstream stages of development, it’s vital that any data provided to an algorithm for training is data that you understand in all its complexity. “There are many ‘flavors’ of data quality,” Moor explains. “It’s important to know how the raw data was generated—why were certain measurements collected? During routine care, it’s often the case that a measurement (such as a lab test) contains a lot of ‘sampling information’ from a doctor who already suspects that something is wrong with the patient. For example, if a patient receives a lactate measurement in the ICU, chances are that clinicians already think the patient may have a critical condition like sepsis, making early warning systems for sepsis less valuable if they were trained—and thus rely heavily—on measurements that are only available after clinicians suspect the condition.”
Celi also cautions against the impulse to provide algorithms with only clean data during development. “The preoccupation with clean, ‘high-quality’ data to build AI is a recipe for disaster,” he says. “If one only develops algorithms from such high-quality data, the algorithm’s failure in the real world is guaranteed. Ideally, algorithms are developed on ‘dirty’ data because they will be deployed on dirty data in real time.”
Considerations for the clinical lab
What can clinical lab professionals do to assess and mitigate bias in the AI tools they develop and use?
First, assess the training datasets of any algorithms that will potentially be incorporated into the lab’s workflows. “Low-hanging fruit for detecting bias in a clinical dataset is to check whether certain groups (such as minority groups) differ in any way in how they received care—what measurements (tests, monitoring, and so on) were performed when (how early, how late, how often)?” Moor explains. Ideally, training data shouldn’t be derived from a single institution and should combine various datasets to ensure that important variables such as race, ethnicity, language, culture, and social determinants of health are captured and included to minimize bias.4
Next, labs should make sure that datasets are representative of and specialized to the given system or setting in which they wish to deploy the algorithm.5 “Usually, it’s hard to generalize how well a given system or solution works in settings that are different from the development and training environment—unless put to the test,” Moor says. “We previously found that specialized models for sepsis prediction may generalize across country borders in an international multicenter evaluation.6 For more general models, studying generalization is much harder, because hundreds—if not thousands—of tasks and aspects may need to be rigorously tested to make such claims (compared to specialized models).”
Additionally, Moor advises considering who will benefit from a given system or solution. “This is especially important because the healthcare business landscape is usually not directly aligned with patient welfare, so it may be naïve to assume that technologies pursued by big players like insurers are here to improve patient care, rather than for profit. I believe there is a certain onus on developers of medical AI systems to be mindful about how certain systems or applications may be used downstream and whether or not patients or caregivers will experience a net benefit.”
Finally, consider AI debiasing another area in which the laboratory can collaborate with other stakeholders. “On their own, clinical lab professionals will not be able to detect biases in their datasets,” says Celi. “They need to work with a diverse team from across disciplines, including patients and their caregivers.”
References:
-
- A Arora et al. The value of standards for health datasets in artificial intelligence-based applications. Nat Med. 2023;29(11):2929–2938. doi:10.1038/s41591-023-02608-w.
-
- M Mittermaier et al. Bias in AI-based models of medical applications: challenges and mitigation strategies. NPJ Digit Med. 2023;6(1):113. doi:10.1038/s41746-023-00858-z.
-
- Y Yang et al. The limits of fair medical imaging in AI in real-world generalization. Nat Med. 2024; online ahead of print. doi:10.1038/s41591-024-03113-4.
-
- LH Nazer et al. Bias in artificial intelligence algorithms and recommendations for mitigation. PLOS Digit Health. 2023;2(6):e0000278. doi:10.1371/journal.pdig.0000278.
-
- J Futoma et al. The myth of generalisability in clinical research and machine learning in healthcare. Lancet Digit Health. 2020;2(9):e489–e492. doi:10.1016/S2589-7500(20)30186-2.
-
- M Moor et al. Predicting sepsis using deep learning across international sites: a retrospective development and validation study. EClinicalMedicine. 2023;62(1):102124. doi:10.1016/j.eclinm.2023.102124.
Subscribe to Clinical Diagnostics Insider to view
Start a Free Trial for immediate access to this article