Computing the Future of the Lab
Computational biology expert Keaun Amani shares his advice and insights on AI and machine learning tools for the clinical lab
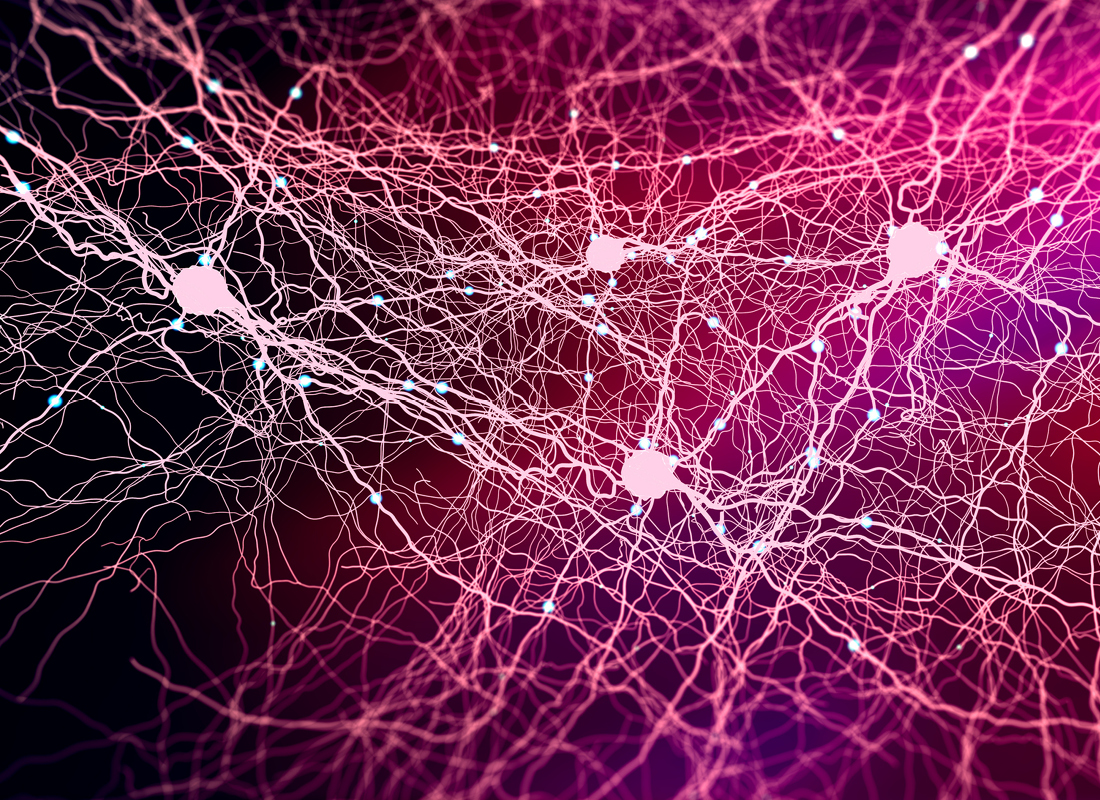
The artificial intelligence (AI) hype is at an all-time high—no less so in the lab than in any other field. But applying these technologies to clinical lab data is a challenge that requires careful consideration, especially in areas where the algorithms still have significant limitations. We spoke to Keaun Amani—software engineer, synthetic biologist, and founder and CEO of Neurosnap, a company focused on machine learning for research and clinical lab applications—to learn more about where lab AI stands today and where it may go next.
Q: What is the current state of AI in the lab?
A: AI and machine learning (ML) models have been in a biomedical renaissance period recently. Every few weeks, we see innovations that push the boundaries in the life sciences and medicine.
A great example is the advent of convolutional neural networks, which specialize in computer vision tasks humans find challenging. Cell segmentation is one such task; annotating individual cells involves determining the boundaries between cells, which isn’t always easy for the human eye. Tasks like this can be applied in a variety of settings, including clinical trials, so I think the trend of increasing AI support will certainly continue.
My personal prediction is that we’ll see a lot more AI models explicitly trained on multiomic data—that is, genomic, proteomic, metabolomic, and even lipidomic data. This will play a big role in shaping the future of ML models in the lab.
Q: How is AI improving our ability to work with clinical lab data?
A: Each generation of AI models helps us develop, validate, and improve the next. Many of the more sophisticated models these days were trained by other models or using their own previous work to improve their accuracy and predictive power. For example, when creating AlphaFold 2, developers took protein structures the model had already predicted and used those to retrain and improve it. This is something I see happening more and more.
AI can also automate triage—for instance, by evaluating slides or other diagnostic images to determine which should be prioritized for human review. My opinion is that AI’s job isn’t to replace humans; it’s to help humans with tedious tasks. For example, an AI model might take raw diagnostic images and identify those most likely to contain signs of disease—or even highlight specific areas of concern for human pathologists to examine in detail. It’s not removing the pathologist from the pipeline; it’s making the pathologist’s job easier and ensuring that the most urgent cases receive the rapid attention they need.
Q: What can’t AI do well at the moment?
A: You may have seen the trend of people failing to get transformer-based models like ChatGPT to accurately state how many Rs are in the word “strawberry.” The underlying reason is that these models don’t perceive individual letters; instead, words and sentences are broken up into segments called tokens. So instead of seeing the word “strawberry,” the model actually sees a number of tokens that combine to form the word—which means that the word itself, and the letters within it, have little meaning. If your models use any kind of tokenization process—as many scientific and medical AI tools do—you need to make sure those nuances don’t become a problem.
Another challenge is the context window, which is the amount of information an AI model can process at once. If you have a long session with ChatGPT, you might notice that it starts to forget certain pieces of information. That means the total volume of information is too great and therefore some of it falls outside the model’s context window. To the model, that information no longer exists. This creates problems in science and medicine. Genomes, for instance, are huge—so if you’re training a model on genomic data, you need an extremely long context window. If you can’t capture all of the necessary data at once, you might lose valuable information, miss important connections, or generate inaccurate summaries.
Finally, there’s a significant lack of high-quality data for training models to analyze images. You may have a very large dataset, but the data might contain a lot of noise, missing pixels, poor lighting, or other issues. As a result, models trained on that dataset will not perform well. Curating high-quality datasets and ensuring that they are used to accurately train scientific and medical AI models is key to the future of AI in the lab.
Q: What do clinical lab professionals need to know about AI?
A: If you’re going to use an AI model, you should know its source. Where is it being offered? Where is it being used? If the model is widely used, commercially available, or provided by an academic lab, start by going through all of its documentation and ask as many questions as possible. Don’t be shy. At the end of the day, the models you use could have very important implications for research or patient care—and may have crucial caveats you need to know to enter data or interpret outputs correctly—so doing your homework is a must.
Additionally, make sure you understand exactly what input your model is trained to use. Humans are much smarter than AI models. We can adapt to seeing data in slightly different formats, but if AI tools aren’t given data in the specific format on which they’ve been trained, they may not be able to interpret it at all.
Q: What should lab leaders and administrators know?
A: If you’re considering bringing these technologies into your lab, do it. They can be absolutely transformative when adopted properly.
Always test the models you’re considering on your own data. If you’re automating a task your lab has been performing manually for a long time, chances are you already have input data and an idea of the expected output. Whenever I assess a new protein structure prediction model, I test it using two sets of proteins: those that existing models fold easily and those with which they struggle. It takes some time, but it’s a helpful gauge of performance—and I think the time investment is worthwhile for a tool that could transform your entire workflow.
It’s also worth evaluating the cost-benefit ratio. A good platform shouldn’t be excessively expensive these days unless it’s doing something truly novel, but it can accelerate or automate processes that might otherwise take months or cost millions. Embracing AI in the lab may seem daunting at first—but, if you approach it with an open mind, it can pay huge dividends down the line.
Subscribe to Clinical Diagnostics Insider to view
Start a Free Trial for immediate access to this article