Lab Data’s New Role as the Backbone of AI-Driven Clinical Trials
Learn how research and development labs can leverage AI to transform laboratory data into actionable insights
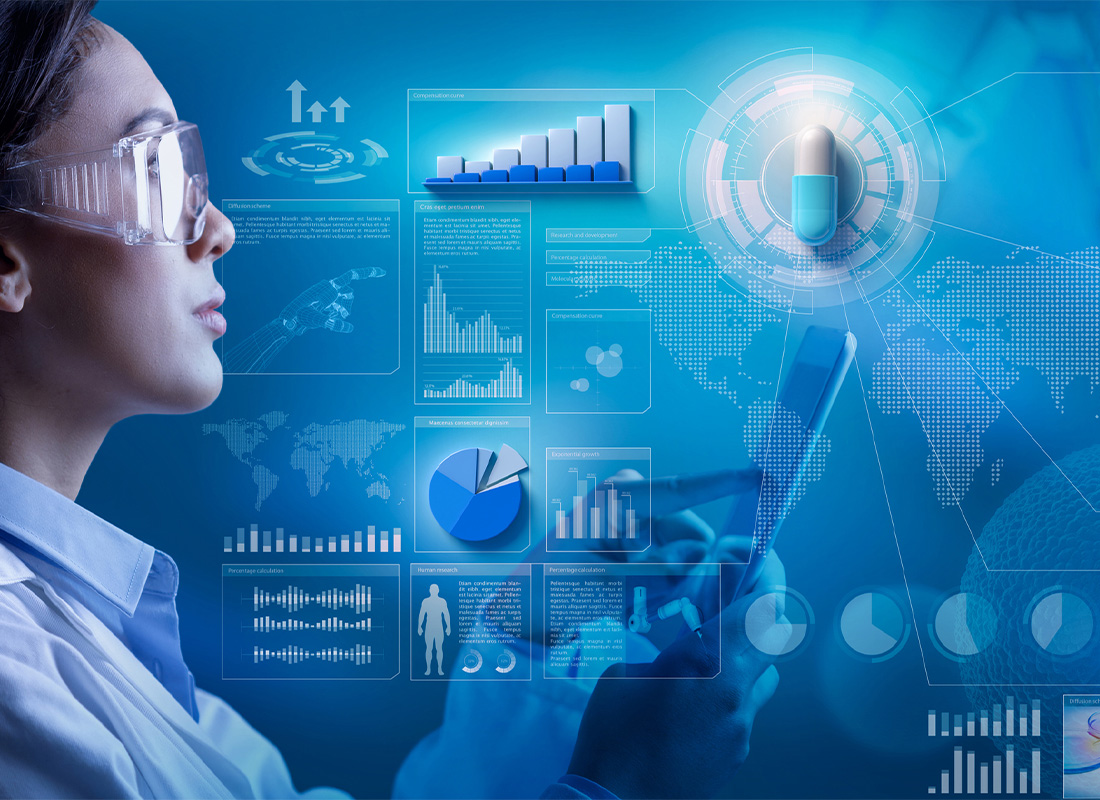
Clinical trials remain fraught with inefficiencies and high failure rates. On average, 90 percent of clinical trials fail to bring a drug to market, often due to poor design or barriers to selecting ideal participants for the aims of the trial.1 These failures not only delay life-saving treatments but also burden the healthcare system with escalating costs.
However, by pairing lab data with artificial intelligence (AI), the clinical trial process is being transformed. Recent breakthroughs, such as AI applications in cancer pathology reports to measure disease severity, demonstrate the potential of this synergy.2 These progressions may result in streamlined processes, reduced costs, and improved outcomes, paving the way for advancements in the design and implementation of clinical trials.
Lab Data is an Underutilized Treasure
What is lab data in the clinical context?
In clinical trials, lab data encompasses a diverse range of information, including pathology reports, genetic sequences, biomarker profiles, and biochemical analyses. Historically, this data has been siloed, serving primarily diagnostic or confirmatory roles rather than driving trial innovation.3
From diagnostics to clinical trials
Traditionally, lab data has been underutilized in clinical trials due to the complexity of integrating diverse datasets.4 The application of these various datasets was limited, focusing more on confirming patient eligibility rather than influencing trial design or execution. But with the rise of AI, the tide is turning.
Unlocking lab data’s potential
Today, lab data is essential to enhancing clinical trials. Lab data can be used to identify the right patient populations, refine trial protocols, and enable real-time monitoring. Researchers can now harness lab data at a larger scale than previously possible. This ability offers many opportunities to reduce inefficiencies and increase the chances of success.
Real-World Implementation of AI in Clinical Trials
Cancer severity prediction
AI tools are now capable of analyzing pathology reports to measure cancer severity, providing a level of precision that was previously unattainable. By using machine learning algorithms, these tools can stratify patients more effectively, ensuring that trials enroll individuals who are most likely to benefit.5 This could result in:
- Faster patient recruitment
- Improved stratification of data, improving possible trial outcomes
- More cost savings by minimizing trial delays
Optimizing patient selection
The combination of genetic and biomarker data with AI has revolutionized patient selection. For instance, AI algorithms can match participants to trials by identifying specific genetic markers or biomarker profiles, ensuring that only the most suitable candidates are enrolled.6 This reduces recruitment time, a major bottleneck in clinical trials, while improving trial efficacy.
Real-time monitoring and protocol adjustments
AI-driven tools are enabling real-time analysis of lab data during trials.7 These tools can flag safety risks, identify adverse trends, and suggest adjustments to trial protocols on the fly. This may boast numerous benefits for future clinical trial execution, including:
- Enhanced flexibility in trial design
- Reduced risk to participants
- Improved data quality and patient outcomes
Challenges and Regulatory Considerations
Despite its transformative potential, the integration of AI and lab data into clinical trials poses many challenges that clinicians and researchers must work together to solve.
Data integrity and compliance
Adhering to Good Laboratory Practice (GLP) is critical. Ensuring that lab data remains accurate, reliable, and secure is paramount for regulatory compliance. Any lapse in data integrity can jeopardize trial outcomes and delay drug approval.
Validation of AI tools
Before deploying AI in clinical trials, rigorous validation is required. Regulatory agencies, including the FDA and EMA, are increasingly scrutinizing AI algorithms to ensure their reliability and accuracy. Developers must demonstrate that AI tools meet stringent performance standards. For example, digital health technologies (DHTs) and decentralized designs offer potential in streamlining clinical trials, and the FDA has established a steering committee to guide their application and implementation.8
Evolving regulatory landscape
Regulatory bodies are beginning to issue guidance on the use of AI in clinical trials.9 This includes frameworks for AI application to clinical trials, data security, and ethical considerations.10 Staying abreast of these changes will be critical for organizations looking to leverage AI effectively.
Trends in Lab Data and AI
The future of clinical trials appears to lie in the continued evolution of AI and lab data. There are several emerging trends that laboratorians should be aware of.
Federated learning
This approach allows collaboration across institutions without the need to share raw data. By training AI models on distributed datasets, federated learning enhances privacy while enabling robust insights.11
AI-driven trials simulations
Simulating trial outcomes before execution can reduce trial failure rates. AI can model various scenarios based on lab data, helping researchers refine protocols and improve the likelihood of success.12
Expansion into personalized medicine
Lab data and AI are pivotal for personalized medicine, enabling the design of hyper-specific trials tailored to individual patients. This shift promises to deliver treatments that are not only more effective but also safer.13
A Call to Action
The integration of lab data and AI into clinical trials is not just a technological advance; it is a paradigm shift with the potential to revolutionize how we develop treatments. By leveraging these tools, the industry can reduce costs, accelerate timelines, and ultimately improve patient outcomes.
To realize this vision, laboratories and clinical trial organizations must:
- Invest in AI technologies: Equip teams with cutting-edge tools to process and analyze lab data at scale
- Train staff in advanced analytics: Build in-house expertise to bridge the gap between data science and clinical research
- Forge partnerships with AI innovators: Collaborate with tech leaders to stay at the forefront of innovation
The potential of AI and lab data is immense. By fully embracing this innovation, clinical trials can deliver faster and more effective treatments to the patients who need them most. When implemented thoughtfully, AI has the power to make clinical trials not only more efficient but also considerably more successful.
References:
- https://pmc.ncbi.nlm.nih.gov/articles/PMC9293739/
- https://www.nature.com/articles/s41467-024-53190-9
- https://pmc.ncbi.nlm.nih.gov/articles/PMC9012601/
- https://www.sciencedirect.com/science/article/abs/pii/S074756322400089X
- https://www.cedars-sinai.org/newsroom/new-ai-method-measures-cancer-severity-using-pathology-reports/
- https://pmc.ncbi.nlm.nih.gov/articles/PMC10088085/
- https://www.nature.com/articles/d41586-024-00753-x
- https://www.fda.gov/drugs/news-events-human-drugs/role-artificial-intelligence-clinical-trial-design-and-research-dr-elzarrad
- https://www.fda.gov/science-research/focus-areas-regulatory-science-report/focus-area-artificial-intelligence
- https://www.fda.gov/science-research/advancing-regulatory-science/evaluate-application-artificial-intelligence-adaptive-enrichment-clinical-trials
- https://research.ibm.com/blog/what-is-federated-learning
- https://www.forbes.com/sites/greglicholai/2024/10/10/ai-simulations-help-drug-trials/
- https://pmc.ncbi.nlm.nih.gov/articles/PMC7580505/
Subscribe to Clinical Diagnostics Insider to view
Start a Free Trial for immediate access to this article