Has Your AI Been Validated?
Many artificial intelligence-based medical devices have not undergone clinical validation—but what does this mean for the lab?
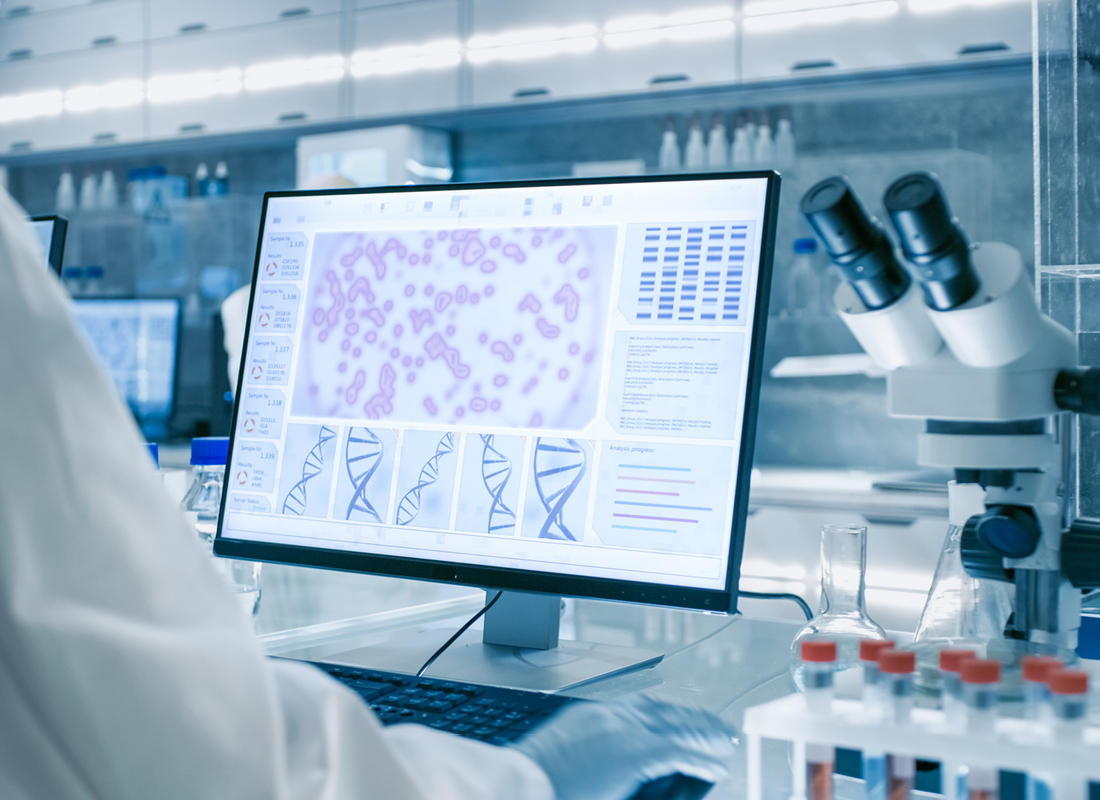
No physician would implant a pacemaker or insulin pump into a patient without ensuring that the device had been thoroughly tested in the clinic first. Although it seems obvious that medical devices should be clinically validated to receive regulatory authorization, that’s not always the case—particularly when it comes to artificial intelligence and machine learning (AI/ML)-based tools.
At the moment, the U.S. Food and Drug Administration (FDA) considers AI algorithms “software as a medical device” and evaluates them in the same way as it would a physical device1—but, despite the organization’s statement that “devices that rely on AI/ML are expected to demonstrate analytical and clinical validation,” not all submissions require clinical data.2 For example, many AI tools submitted for market authorization via the 510(k) premarket submission pathway can use non-clinical data to demonstrate substantial equivalence to existing tools. Even when clinical validation is required, there is no clear guidance as to what form that clinical data may take—for instance, in determining whether retrospective data will suffice or whether a prospective trial is needed.3
The value of validation
Clinical validation of AI-based medical devices is crucial for many reasons. “AI models require great amounts of data to learn, improve, and perform their functions effectively,” says Sammy Chouffani El Fassi, a researcher at Duke University Hospital and first author of a recent paper examining rates of clinical validation in medical AI. “Data collection at the scale of health systems draws concern to patient privacy. The math involved in AI is sometimes so complex that humans cannot understand how or why it produced a result, a scenario termed the ‘black box problem.’ Furthermore, implementing a device that is not proven to work well can waste time and money. These are just some of the many reasons that clinical validation is important.”
Chouffani El Fassi and his colleagues found that, of the 521 medical AI devices authorized by the FDA between 1995 and 2022, only 56 percent had been evaluated for safety and effectiveness using real patient data. Additionally, just over 4 percent of all authorized devices had been evaluated using a randomized controlled trial.4
This is particularly concerning alongside another recent study showing that GPT-4V, a large language model (LLM) with image processing capabilities, achieved less than 48 percent accuracy in answering image-based multiple-choice questions from radiology board examinations.5 The model also showed a tendency to hallucinate. When it did provide correct answers, they were sometimes based on incorrect image interpretations.
“These findings underscore the need for more specialized and rigorous evaluation methods to accurately assess the performance of [LLMs],” the authors wrote in their paper.5 “Enhanced assessment approaches may offer a deeper understanding of LLMs’ analytical processes and limitations in clinical contexts.”
Setting the standard
No standard currently exists for clinical validation of medical AI; in fact, Chouffani El Fassi’s paper highlights the lack of not only guidance, but even a common language for discussing the necessary concepts. To address this, the authors propose simple definitions that cover a range of clinical validation methods:
- Clinical validation: the device was tested with real patient data to evaluate its safety and effectiveness.
- Prospective validation: the device was tested after implementation in patient care, or data were collected after the study began.
- Retrospective validation: the device was tested before implementation in patient care, or data were collected before the study began.
“We do not know what challenges may be involved in publishing more clinical validation data, nor do we know the consequences of requiring clinical validation data for a greater proportion of device authorizations,” says Chouffani El Fassi. “This is why we have not been particularly critical of the FDA. Rather, we argue that, given device risk and the potential for distrust to hinder adoption, ample clinical validation data must be published for the public to accept FDA authorization as an indication of device effectiveness.”
Who needs clinical validation?
AI experts are quick to point out that, although many devices would benefit from clinical validation, it’s not always necessary—or possible.
“There are three possible scenarios,” says Keaun Amani, a software engineer and synthetic biologist whose company, Neurosnap, develops AI models for research and clinical use. “One is that it’s simply not viable to test a new AI model on clinical data because the necessary clinical data don’t exist yet. The second is that the model’s intended function doesn’t need clinical validation, so there’s no benefit to that testing. And the third is that the model would, in fact, benefit from validation on existing clinical data but, for whatever reason, it wasn’t trained or tested on those data.”
Should clinical lab professionals be concerned about adopting AI tools to streamline their work, given the possibility of Amani’s third scenario? Not necessarily, he says—it depends on the potential consequences of using that model. If designed to assist with disease diagnosis or monitoring, an unreliable AI model poses an unacceptable level of risk; for other purposes, such as formatting reports or helping phrase patient messages, the tolerance for error or uncertainty may be higher.
“If you see a model or a pipeline that is highly applicable to your lab’s workflow, then I would absolutely recommend spending the time and money to investigate that model,” says Amani. “You want to ensure that it works well not just in general, but for your specific purposes. When organizations are considering adopting our models, they supply us with datasets they use internally and we give them the outputs our models generate. That allows them to assess whether or not the models are a good fit for their work.”
Although it’s important for clinical labs to perform their own evaluations of AI tools before trusting them as essential components of the lab workflow, accurately judging a model’s capabilities can be difficult without clinical validation data. For that reason, Chouffani El Fassi and his colleagues are calling for more thorough studies of new AI-based tools as part of the regulatory approval process.
“What we say to all stakeholders—device manufacturers, universities, researchers, and regulatory bodies—is: conduct the clinical validation studies, use our standard to evaluate the strength of the clinical validity, and report the data to the public,” says Chouffani El Fassi. “This will facilitate trust, accelerate adoption, protect patients, and lead to the development of higher-quality technology.”
References:
- U.S. Food and Drug Administration. Software as a Medical Device (SaMD). December 4, 2018. https://www.fda.gov/medical-devices/digital-health-center-excellence/software-medical-device-samd.
- U.S. Food and Drug Administration. Proposed Regulatory Framework for Modifications to Artificial Intelligence/Machine Learning (AI/ML)-Based Software as a Medical Device (SaMD). April 2, 2019. https://www.fda.gov/media/122535/download.
- U.S. Food and Drug Administration. Recommendations for the Use of Clinical Data in Premarket Notification [510(k)] Submissions: Draft Guidance for Industry and Food and Drug Administration Staff. September 7, 2023. https://www.fda.gov/media/171837/download.
- Chouffani El Fassi S et al. Not all AI health tools with regulatory authorization are clinically validated. Nat Med. 2024; online ahead of print. doi:10.1038/s41591-024-03203-3.
- Hayden N et al. Performance of GPT-4 with vision on text- and image-based ACR Diagnostic Radiology In-Training Examination Questions. Radiology. 2024;312(3):e240153. doi:10.1148/radiol.240153.
Subscribe to Clinical Diagnostics Insider to view
Start a Free Trial for immediate access to this article