LLMs and the Lab
How artificial intelligence models can help labs, clinicians, and patients communicate with one another more effectively
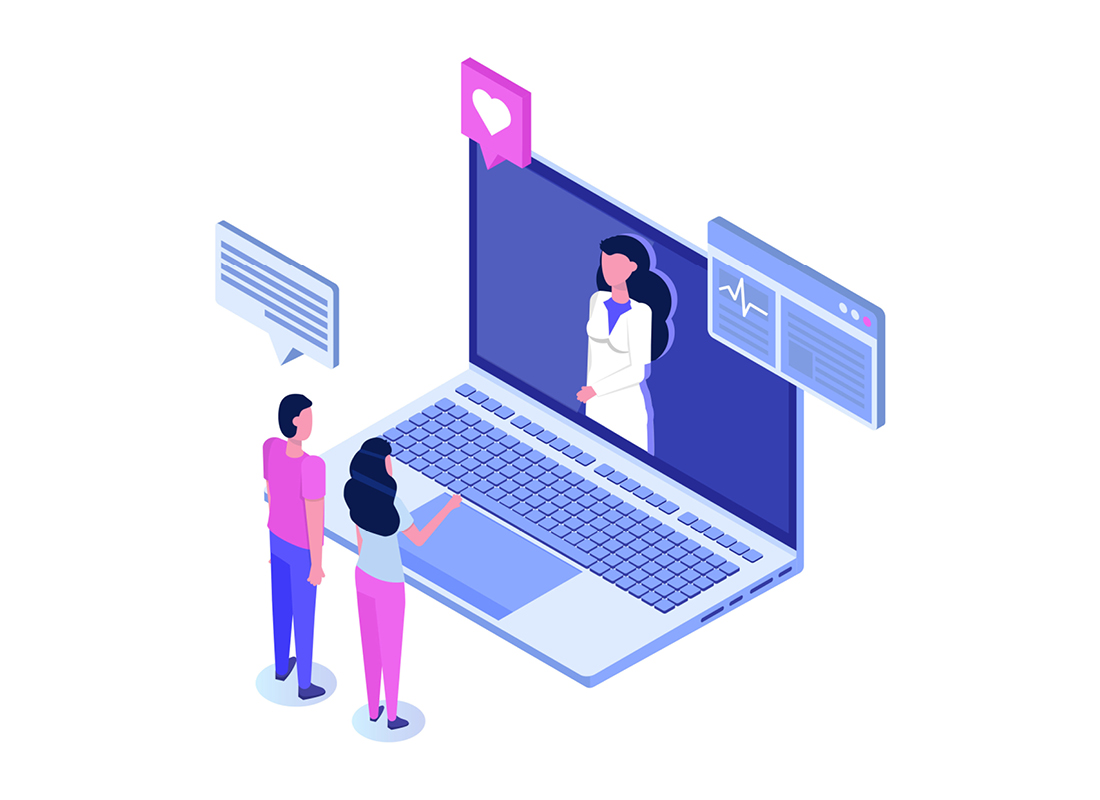
Despite increasing awareness of the lab’s involvement in healthcare, many patients still don’t understand what goes on behind closed doors. Test results, though available to patients as a result of the 21st Century Cures Act and similar reforms,1 are often phrased in complex ways and use technical jargon that makes them incomprehensible to non-experts. When combined with barriers such as poor health literacy2 or a lack of technological proficiency,3 it’s not difficult to see why patients struggle to connect with the lab or value its services. Could artificial intelligence (AI) tools help? Pathologists and patient experts think so—and they’re eager to explore the possibilities.
Readable reports
Why make laboratory results more accessible? “Informed clinicians and patients make the right decisions,” says John Groth, an anatomic and clinical pathologist at Endeavor Health Medical Group. “The assumption is that clinicians know what we’re saying—but we’ve found that that isn’t always true. Now, we’re looking to see how large language models (LLMs) can help clinicians understand and explain lab results.” Research has shown that LLM assistance can improve clinicians’ diagnostic accuracy over standard supports like search engines and textbooks.4
Studies have also demonstrated that patients have a high level of interest in pathologist consultations5 and a strong sense of empowerment after being shown their slides and given the opportunity to better understand their diagnoses.6 That’s why Groth and his colleagues are working on creating an LLM module to help patients engage with their lab results. “I’ve been doing pathology clinics and seeing patients for over 12 years—but not every patient is going to want to see the pathologist,” Groth explains. “LLMs offer an alternative. And it’s not just about interpretation; it’s also about combining information in multimodal ways—translating it into multiple languages, creating videos, and so on. We want to give patients the option to say, ‘How do I want this information? Do I want text, images, or video?’”
Matthew Hanna, vice chair of pathology informatics and director of AI operations at the University of Pittsburgh Medical Center, supports efforts to bring information to patients—but cautions that AI tools need further development. “There should be pathologist oversight of patient-facing applications,” he says, citing a study in which he and his colleagues evaluated AI chatbots’ ability to interpret pathology reports for patients.7 The tools significantly decreased the complexity of the reports, but errors and hallucinations persisted, leading to his recommendation that human experts review the simplified text before providing it to patients.
Groth agrees. “These tools can’t replace pathologists,” he says. “They enhance and augment pathologists’ work.”
The patient perspective
“Recently, I had a health scare and thought my breast cancer might have returned,” says patient advocate Michele Mitchell. “I was anxiously awaiting the pathology report and, when it arrived, I immediately went to Google and ChatGPT to analyze what it said. There was nothing more important at that point in my life than knowing whether or not the cancer was back.”
Many patients feel the same sense of urgency when awaiting potentially life-changing results, which is why LLMs are a growing presence on the healthcare stage. “People want answers and they will do whatever they need to get them. Do I have a condition or don’t I? What does my result mean? I know that we need to be concerned about hallucinations and errors, but the reality is that people will go out and look for answers.”
Mitchell is equally enthusiastic about clinical lab professionals’ use of AI tools. “As a patient, I would feel comforted in knowing that my biopsies and test results are run through AI to confirm the diagnosis. I assume it would potentially help eliminate misdiagnoses as well, so I would find it comforting to know that no stone was left unturned.” Not only that, but LLMs can help laboratorians condense their results and reports into summaries that the average patient can read and understand,8 facilitating communication between labs, clinicians, and patients.
“As part of the Cures Act, patients now have access to their slide images and test results,” Groth points out. “If we haven’t used AI, the patients will do it themselves and then come back to us and say, ‘What do you think?’ In my opinion, it will be a driver for adoption of AI technologies in the clinical lab.”
The ground truth . . .
With respect to the errors and hallucinations LLMs may produce, Groth says, “What is ground truth? I think these technologies challenge our current concepts of ground truth and how pathologists’ interpretations and consensus relate to it.”
In pathology, ground truth—the standard reference data used to teach AI models what is “correct”—consists of a consensus diagnosis between human experts. This leaves the door open for subjectivity in terms of result interpretation, disease characterization, the gravity of potential diagnostic error, and more. “I don’t think we have any way around that,” says Hanna. “My concerns are not just the potential for hallucinations or errors, but also that the performance evaluation aspect itself is challenging—so it’s harder to know whether an LLM did a good job at scale.”
. . . and the blue-sky future
“I use ChatGPT all the time,” says Mitchell. “My husband just had an unusual test done and the results came back a couple of weeks before his next appointment, so I put them into ChatGPT and it came back with explanations that made me much more comfortable waiting.” She encourages other patients to use the resources available to them, but also recommends that clinical lab professionals consider the potential benefits of AI in helping them write patient-legible summaries, reply to questions, or even raise awareness of the lab’s value among non-experts.
The challenge facing labs now is keeping their knowledge of AI up to date. Hanna says, “The speed at which everything is being updated is unprecedented. The field specifically around generative AI is changing so rapidly that what’s state-of-the-art today will become outdated almost as soon as the paper gets published.”
Nonetheless, all three experts agree that patient- and clinician-legible content is vital to effective communication outside the lab—and that, because LLMs are here to stay, embracing their ability to help create that content may offer a promising path forward.
“Embrace the idea of meeting with patients and using these tools,” says Mitchell. “AI can augment your job—and I think it’s going to redefine the field of pathology and laboratory medicine.”
References:
- An Act to accelerate the discovery, development, and delivery of 21st century cures, and for other purposes. One Hundred Fourteenth Congress of the United States of America. January 4, 2016. https://www.congress.gov/114/bills/hr34/BILLS-114hr34enr.pdf.
- Lazaro G. When positive is negative: health literacy barriers to patient access to clinical laboratory test results. J Appl Lab Med. 2023 Nov 2;8(6):1133–1147. doi:10.1093/jalm/jfad045.
- Zhang Z et al. Patient challenges and needs in comprehending laboratory test results: mixed methods study. J Med Internet Res. 2020;22(12):e18725. doi:10.2196/18725.
- McDuff D et al. Towards accurate differential diagnosis with large language models. arXiv. 2023. doi:10.48550/arXiv.2312.00164.
- Lapedis CJ et al. The patient-pathologist consultation program: a mixed-methods study of interest and motivations in cancer patients. Arch Pathol Lab Med. 2020;144(4):490–496. doi:10.5858/arpa.2019-0105-OA.
- Booth AL et al. “Please help me see the dragon I am slaying”: implementation of a novel patient-pathologist consultation program and survey of patient experience. Arch Pathol Lab Med. 2019;143(7):852–858. doi:10.5858/arpa.2018-0379-OA.
- Steimetz et al. Use of artificial intelligence chatbots in interpretation of pathology reports. JAMA Netw Open. 2024;7(5):e2412767. doi:10.1001/jamanetworkopen.2024.12767.
- Zaretsky J et al. Generative artificial intelligence to transform inpatient discharge summaries to patient-friendly language and format. JAMA Netw Open. 2024;7(3):e240357. doi:10.1001/jamanetworkopen.2024.0357.
Subscribe to view Essential
Start a Free Trial for immediate access to this article