Pandemics: Prediction, Prevention, and Response
Recent efforts to forecast the next infectious disease outbreak and steps labs can take to ensure they’re prepared.
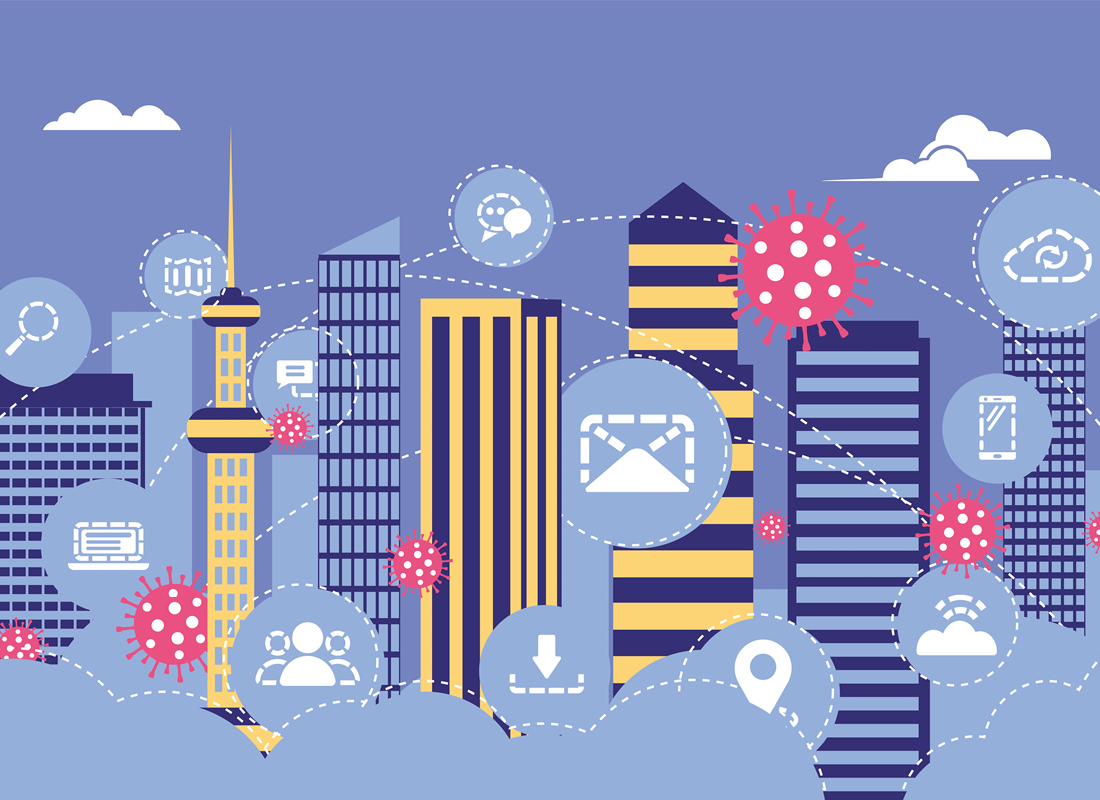
After the events of 2020, clinical laboratories are acutely aware of the potential impact of future infectious disease outbreaks. Many are still recovering from the effects of COVID-19, and most have seen changes in everything from training opportunities1 to workplace risk assessments.2 In response, numerous efforts to predict and prevent future pandemics have arisen—but how can clinical laboratories support these efforts, and what can they do to be ready for the next threat?
Learning from the past
Which pathogens have pandemic potential? Several characteristics contribute to a pathogen’s threat level: high transmissibility, asymptomatic spread, lack of preexisting immunity, and microbial factors that increase virulence or immune evasion.3 Focusing on transmissibility and human infectivity, researchers in the UK analyzed the genomes of 743 RNA viruses to look for changes over time.4 They found that human-infective and strictly zoonotic viruses evolve separately, but both arise from nonhuman-infective ancestors. Furthermore, most viruses capable of epidemic spread in humans emerge from nonhuman reservoirs—suggesting that these sources should be targeted for pandemic surveillance. “Viruses without the right ancestry don’t seem to cause epidemics,” said lead author Mark Woolhouse, professor of infectious disease epidemiology at the University of Edinburgh, in a recent press release. “Out of potentially huge numbers of mammal and bird viruses in circulation, we should concentrate on the ones that are related to existing human viruses with epidemic potential.”5
Even once a virus’s pandemic potential is known, it can be difficult to predict how it may evade our immune systems, vaccines, and treatments. Consider SARS-CoV-2: only after the emergence of the Omicron variant were scientists able to identify the drivers underlying its evolution and adaptation—including host factors such as immune history and viral characteristics such as tissue preference.6
AI-assisted prediction
To better predict and prepare for future pandemics, we must anticipate new viral mutations and their effects. This need led to the development of EVEscape, an artificial intelligence (AI) tool that combines knowledge of existing viruses and immunity with the ability to predict the viral mutations most likely to offer an evolutionary benefit.7 Currently, the EVEscape team issues a biweekly report on circulating SARS-CoV-2 variants and their immune escape potential; soon, the researchers hope to expand its applications to other pathogens with pandemic potential, such as Lassa and Nipah viruses. Study co-author Sarah Gurev explained, “By rapidly determining the threat level of new variants, we can help inform earlier public health decisions.”8
EVEscape isn’t the only project using AI to get ahead of the next pandemic. Researchers who assembled and examined a ~400-year (1600–2019) dataset of global epidemics highlighted the surprisingly high—and rising—likelihood of “extreme epidemics.”9 With that in mind, numerous research groups are focusing on machine learning technologies to help understand drivers of pathogenicity and disease spread. Two examples are epidemiologically informed neural networks (EINNs)10 and epidemiological priors informed deep neural networks (Epi-DNNs),11 both of which can effectively model outbreaks of diseases like COVID-19 and influenza while avoiding common issues such as restrictions on variables or high data quantity and quality requirements.
Researchers are even investigating ChatGPT’s ability to identify pathogens with pandemic potential, describe the emergence of new infectious diseases, and recommend measures to prevent or halt outbreaks.12 Although general tools like ChatGPT require human guidance, they can rapidly analyze and summarize large amounts of data to support prevention and intervention efforts.
For current pandemics, AI can predict the appearance of new variants that may present diagnostic or treatment challenges. A machine learning model trained on 9 million SARS-CoV-2 genomes identified mutation and transmission patterns that heralded the start of each new wave of infections. Using this data, the model was able to identify highly infectious variants with 86.3 percent accuracy one week after first detection and 90.8 percent accuracy two weeks after first detection.13 Similar models could be applied to a range of other respiratory viruses, offering promise for future pandemics.
But AI predictions are only as accurate as the input data, which is often severely lacking in the early stages of an outbreak. Because so many viruses with pandemic potential arise from nonhuman reservoirs, expanding and accessing biorepositories may yield information lacking in human and model organism studies of disease. The authors of a paper recommending better biorepository use14 point out that serological studies, although common in infectious disease research, rarely archive specimens in biorepositories, and that research tools (such as sequences or secondary antibodies) are often unavailable for non-model organisms. Biorepositories that collect and archive specimens from such organisms could provide insights into a pathogen’s history of evolution and transmission—valuable data for AI models aiming to anticipate its next move.
Planning and preparing
What role does the clinical lab play in readiness for the next outbreak? Infectious disease testing is key to spotting outbreaks early and monitoring their progress. Pathogen sequencing can yield details about an organism’s history, mechanisms of transmission and infection, and even potential future adaptations. Not least, as climate shifts15 and changes in human behavior16 continually alter the landscape of infectious disease, laboratory professionals can advise other healthcare providers and educate the public on outbreak identification, monitoring, and prevention.
Lab readiness must be considered from multiple angles. Key questions include:
- Is your laboratory sufficiently staffed to handle a sudden increase in workload—or, if not, are other measures (such as automation) in place?
- Do you offer staff training and support for mental health considerations such as flexibility, resilience, stress, and burnout?
- Do you have redundant sources for key supplies such as common reagents and other consumables?
- Do you have access to the infrastructure and resources needed to handle a sudden influx of samples and data (for instance, in terms of storage or transport)?
- What precautions does your laboratory have in place for reducing staff members’ risk of exposure to a novel pathogen? Are you prepared to implement emergency remote work policies?
- Do you have a plan for maintaining non-pandemic-related work in an emergency situation?
- What regulatory issues might you encounter in the face of a novel pathogen (for instance, in deploying laboratory-developed tests)? Do you have a plan for addressing these?
- Does your lab have strong connections to other healthcare professionals who may be involved in pandemic response (such as primary care providers or public health officials)?
Responding to a public health emergency presents a challenge for all medical professionals—but with robust planning and resilient personnel, clinical laboratories can lead the way on the road back to normality.
References:
- Kabanova A et al. Effect of the COVID-19 pandemic on laboratory and clinical research: a testimony and a call to action from researchers. Hemasphere. 2020;4(6):e499. doi:10.1097/HS9.0000000000000499.
- Jackson BR et al. The lab must go on. Am J Clin Pathol. 2021;155(1):4–11. doi:10.1093/ajcp/aqaa187.
- Adalja AA et al. Characteristics of microbes most likely to cause pandemics and global catastrophes. Curr Top Microbiol Immunol. 2019;424:1–20. doi:10.1007/82_2019_176.
- Lu L et al. Temporal dynamics, discovery, and emergence of human-transmissible RNA viruses. Mol Biol Evol. 2024;41(1):msad272. doi:10.1093/molbev/msad272.
- University of Edinburgh. Virus ancestry could help predict next pandemic. February 5, 2024. https://www.ed.ac.uk/news/2024/virus-ancestry-could-help-predict-next-pandemic.
- Russell TW et al. Combined analyses of within-host SARS-CoV-2 viral kinetics and information on past exposures to the virus in a human cohort identifies intrinsic differences of Omicron and Delta variants. PLoS Biol. 2024;22(1):e3002463. doi:10.1371/journal.pbio.3002463.
- Thadani NN et al. Learning from prepandemic data to forecast viral escape. Nature. 2023;622(7984):818–825. doi:10.1038/s41586-023-06617-0.
- Caruso C. An AI Tool That Can Help Forecast Viral Variants. Harvard Medical School News & Research. October 11, 2023. https://hms.harvard.edu/news/ai-tool-can-help-forecast-viral-outbreaks.
- Marani M et al. Intensity and frequency of extreme novel epidemics. Proc Natl Acad Sci USA. 2021;118(35):e2105482118. doi:10.1073/pnas.2105482118.
- Rodríguez A et al. EINNs: epidemiologically-informed neural networks. Proc AAAI Conf Artif Intell. 2023;37(12):14453–14460. doi:10.1609/aaai.v37i12.26690.
- Ning X et al. Epi-DNNs: epidemiological priors informed deep neural networks for modeling COVID-19 dynamics. Comput Biol Med. 2023;158:106693. doi:10.1016/j.compbiomed.2023.106693.
- Jana PK et al. Predicting future pandemics and formulating prevention strategies: the role of ChatGPT. Cureus. 2023;15(9):e44825. doi:10.7759/cureus.44825.
- Levi R et al. Predicting the spread of SARS-CoV-2 variants: an artificial intelligence enabled early detection. PNAS Nexus. 2024;3(1):pgad424. doi:10.1093/pnasnexus/pgad424.
- Colella JP et al. Advancing the central role of non-model biorepositories in predictive modeling of emerging pathogens. PLoS Pathog. 2023;19(6):e1011410. doi:10.1371/journal.ppat.1011410.
- Vargas-Parada L. Why climate change matters for pandemic preparedness. Nature. 2022;610(7933):S45. doi:10.1038/d41586-022-03359-3.
- Lim TY et al. Why similar policies resulted in different COVID-19 outcomes: how responsiveness and culture influenced mortality rates. Health Aff (Millwood). 2023;42(12):1637–1646. doi: 10.1377/hlthaff.2023.00713.
Subscribe to Clinical Diagnostics Insider to view
Start a Free Trial for immediate access to this article