Tapping AI and Deep Learning to Personalize Breast Cancer Screening for Women
A new study suggests that use of an artificial intelligence (AI) algorithm may provide the key to creating personalized breast cancer screening protocols for women based on the results of their mammograms. The Diagnostic Challenge Early detection reduces breast cancer mortality. While screening mammography helps detect breast cancer early, it is not as effective in breasts with radiologically dense tissue. In addition to being a risk factor, dense tissue exercises a masking effect on detection. This often results in what are called “interval cancers,” i.e., cancers discovered within 12 months after a normal screening mammogram. Such interval cancers account for roughly 13 percent of all breast cancers diagnosed in the U.S. Because these cancers typically have more aggressive tumor biology, interval cancers are often at an advanced stage by the time they are discovered. Because all women are not the same, women at high risk of interval cancer may need more than just routine screenings but also supplemental screenings and other additional prevention measures. The problem with current diagnostics is that they do not identify whether a patient is at high risk. The Study For these reasons, the American College of Radiology has called on researchers to develop direct measures […]
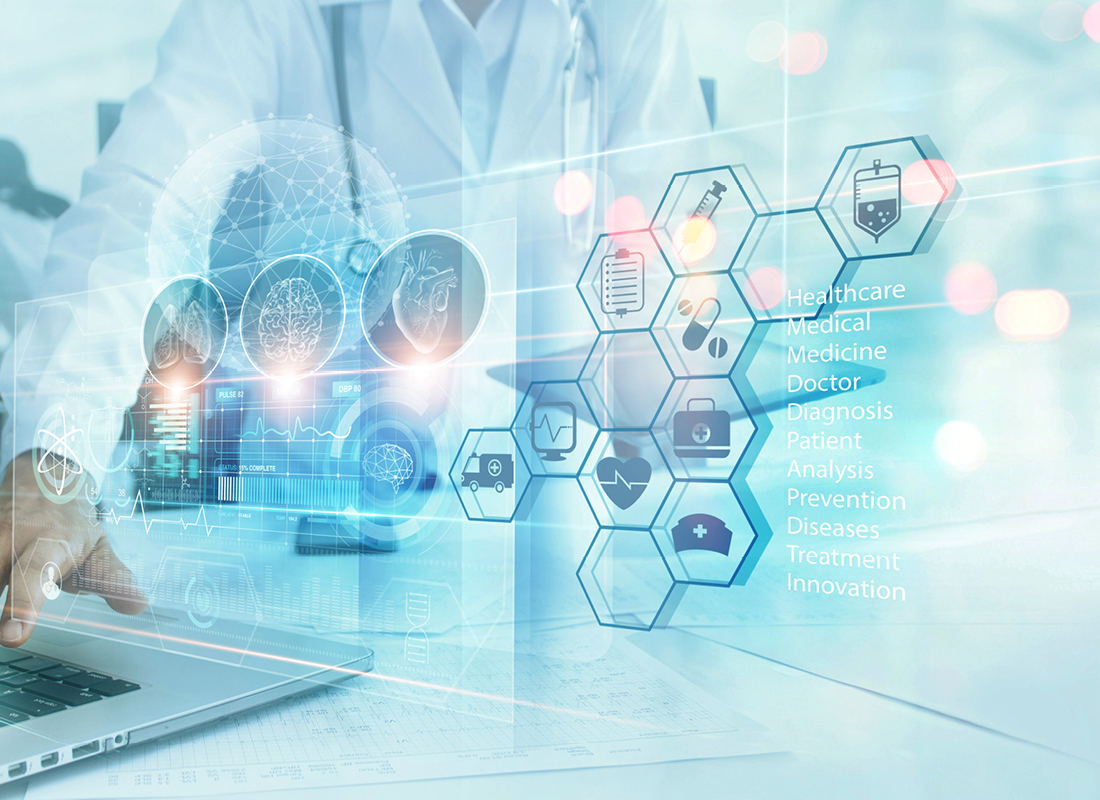
A new study suggests that use of an artificial intelligence (AI) algorithm may provide the key to creating personalized breast cancer screening protocols for women based on the results of their mammograms.
The Diagnostic Challenge
Early detection reduces breast cancer mortality. While screening mammography helps detect breast cancer early, it is not as effective in breasts with radiologically dense tissue. In addition to being a risk factor, dense tissue exercises a masking effect on detection. This often results in what are called “interval cancers,” i.e., cancers discovered within 12 months after a normal screening mammogram. Such interval cancers account for roughly 13 percent of all breast cancers diagnosed in the U.S. Because these cancers typically have more aggressive tumor biology, interval cancers are often at an advanced stage by the time they are discovered.
Because all women are not the same, women at high risk of interval cancer may need more than just routine screenings but also supplemental screenings and other additional prevention measures. The problem with current diagnostics is that they do not identify whether a patient is at high risk.
The Study
For these reasons, the American College of Radiology has called on researchers to develop direct measures of masking and interval cancer risk. Some of the early efforts have focused on applying computer vision methods to mammography using deep learning (DL) and AI.
A few years ago, a team of researchers led by John Shepherd, PhD, of the University of Hawaii Cancer Center in Honolulu completed a study finding that DL shows promise in being able to learn mammographic features beyond density to distinguish between interval and screening-detected breast cancer risk. The new study, which was published online in the Sept. 7 issue of Radiology, builds on that work.
The study was performed on 25,096 digital screening mammograms obtained from January 2006 to December 2013. The mammograms came from 6,369 women without breast cancer, 1,609 of whom developed screening-detected breast cancer and 351 of whom developed interval invasive breast cancer. The researchers trained a DL algorithm model on the negative mammograms to classify women into two groups: those who did not develop cancer, and those who later did develop screening-detected cancer or interval invasive cancer after negative mammograms. Model effectiveness was evaluated as a matched concordance statistic (C statistic) in a held-out 26 percent (1669 of 6369) test set of the mammograms.
The Study’s Findings
The team found that their DL model predicted risk of breast cancer on future screening mammograms better than relying just on clinical risk factors including breast density. The DL model outperformed in determining screening-detected cancer risk but underperformed for interval cancer risk when compared with clinical risk factors including breast density. Specifically, the C statistics and odds ratios for comparing patients with screening-detected cancer versus matched controls were:
- 0.66 (95% CI: 0.63, 0.69) and 1.25 (95% CI: 1.17, 1.33), respectively, for the DL model;
- 0.62 (95% CI: 0.59, 0.65) and 2.14 (95% CI: 1.32, 3.45) for the clinical risk factors with the Breast Imaging Reporting and Data System (BI-RADS) density model; and
- 0.66 (95% CI: 0.63, 0.69) and 1.21 (95% CI: 1.13, 1.30) for the combined DL and clinical risk factors model.
For comparing patients with interval cancer versus controls, the C statistics and odds ratios were:
-
- 0.64 (95% CI: 0.58, 0.71) and 1.26 (95% CI: 1.10, 1.45), respectively, for the DL model;
- 0.71 (95% CI: 0.65, 0.77) and 7.25 (95% CI: 2.94, 17.9) for the risk factors with BI-RADS density (b rated vs non-b rated) model; and
- 0.72 (95% CI: 0.66, 0.78) and 1.10 (95% CI: 0.94, 1.29) for the combined DL and clinical risk factors model.
AI vs. BI-RADS density category for predicting future breast cancer
BI-RADS density category | Deep-learning model | Combined model | |
---|---|---|---|
Distinguishing future screening-detected cancers from control cases | 0.62 | 0.66 | 0.66 |
Distinguishing future interval-detected cancers from control cases | 0.71 | 0.64 | 0.72 |
The P values between the DL, BI-RADS, and combined model’s ability to detect screen and interval cancer were .99, .002, and .03, respectively.
Takeaway
The good news is that the extra signal from AI provided a better risk estimate for screening-detected cancer. This enabled researchers to achieve their goal of classifying women into low risk or high risk of screening-detected breast cancer. Rather than just relying on breast density to guide management decisions and advising women to return next year for another screening, the researchers suggest that practices could use the AI model to categorize women with a negative screening into one of three risk pathways—low risk of breast cancer, elevated screening-detected risk, or elevated interval invasive cancer risk—for the next three years.
“This would allow us to use a woman’s individual risk to determine how frequently she should be monitored,” Shepherd noted. “Lower-risk women might not need to be monitored with mammography as often as those with a high risk of breast cancer.”
The algorithm could also be used to categorize mammograms and decide when to utilize additional imaging modalities. Thus, for example, supplemental MRI, ultrasound, and molecular imaging might be of benefit to women in the high-risk group who have dense breasts and have a higher risk for interval cancers.
“As each individual woman has a different risk for breast cancer, it is possible that women should have personalized screening intervals and tailored options for supplemental screening,” according to one of the study authors.
The bad news is that the AI algorithm did not do as well in predicting interval cancer cases.
Subscribe to Clinical Diagnostics Insider to view
Start a Free Trial for immediate access to this article